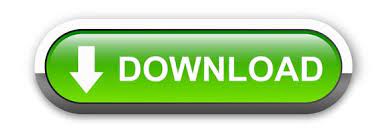
As a result, it makes sense to create an entity around bank account information.Ī number of values might fall into this category of information, such as “username”, “password”, “account number”, and so on. In this example, we’ve been focused on a user’s bank account. Think of nouns, or entities, that surround your intents. Use goals to understand and build out relevant nouns and keywords If your analytical teams aren’t set up for this type of analysis, then your support teams can also provide valuable insight into common ways that customers phrases their questions.ģ. Alternatively, they can also analyze transcript data from web chat conversations and call centers. If your chatbot analytics tools have been set up appropriately, analytics teams can mine web data and investigate other queries from site search data. If you’re unsure of other phrases that your customers may use, then you may want to partner with your analytics and support teams. If we take the example of “how to access my account,” you might think of other phrases that users might use when chatting with a support representative, such as “how to log in”, “how to reset password”, “sign up for an account”, and so on. Once you outline your goals, you can plug them into a competitive conversational AI tool, like Watson Assistant, as intents.įrom here, you’ll need to teach your conversational AI the ways that a user may phrase or ask for this type of information. Your FAQs form the basis of goals, or intents, expressed within the user’s input, such as accessing an account. Use FAQs to develop goals in your conversational AI tool You can always add more questions to the list over time, so start with a small segment of questions to prototype the development process for a conversational AI.Ģ.

If you don’t have a FAQ list available for your product, then start with your customer success team to determine the appropriate list of questions that your conversational AI can assist with.įor example, let’s say you’re a bank. They help you define the main needs and concerns of your end users, which will, in turn, alleviate some of the call volume for your support team.
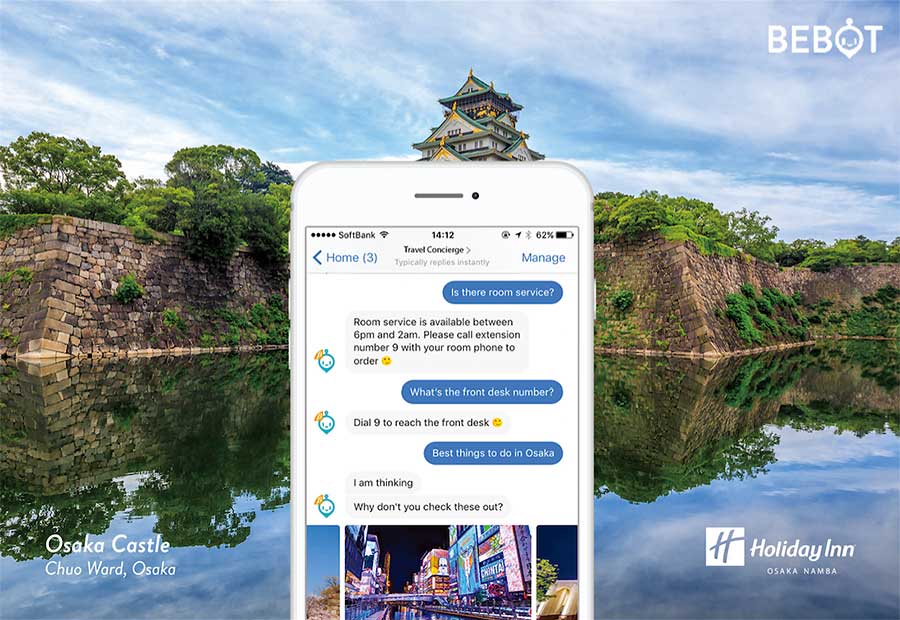
Find the list of frequently asked questions (FAQs) for your end usersįrequently asked questions are the foundation of the conversational AI development process. In this section, we’ll walk through ways to start planning and creating a conversational AI.ġ. You can then use conversational AI tools to help route them to relevant information. Reinforcement learning: Finally, machine learning algorithms refine responses over time to ensure accuracyĬonversational AI starts with thinking about how your potential users might want to interact with your product and the primary questions that they may have.Dialogue management: During this stage, Natural Language Generation (NLG), a component of NLP, formulates a response.However, if the input is speech-based, it’ll leverage a combination of automatic speech recognition (ASR) and NLU to analyze the data. Input analysis: If the input is text-based, the conversational AI solution app will use natural language understanding (NLU) to decipher the meaning of the input and derive its intention.Input generation: Users provide input through a website or an app the format of the input can either be voice or text.These four NLP steps can be broken down further below: Underlying ML algorithms improve response quality over time as it learns. Unstructured data transformed into a format that can be read by a computer, which is then analyzed to generate an appropriate response. NLP consists of four steps: Input generation, input analysis, output generation, and reinforcement learning. In the future, deep learning will advance the natural language processing capabilities of conversational AI even further. Before machine learning, the evolution of language processing methodologies went from linguistics to computational linguistics to statistical natural language processing. Natural language processing is the current method of analyzing language with the help of machine learning used in conversational AI. As the input grows, the AI platform machine gets better at recognizing patterns and uses it to make predictions.

Machine Learning (ML) is a sub-field of artificial intelligence, made up of a set of algorithms, features, and data sets that continuously improve themselves with experience. Conversational AI has principle components that allow it to process, understand, and generate response in a natural way. These NLP processes flow into a constant feedback loop with machine learning processes to continuously improve the AI algorithms. Conversational AI combines natural language processing (NLP) with machine learning.
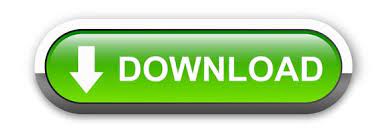